From GIM International
By Kevin Corbley
Canada’s Pollutant Inventories and Reporting Division (PIRD) faces the massive task of creating a greenhouse gas inventory for the entire nation. A key part of this analysis is the use of remotely sensed data to quantify areas of land-use change to calculate CO2. In this pursuit, PIRD has partnered with PCI Geomatics in a project that leverages Open Data Cube technology to enhance the efficiency, accuracy and granularity of analysing enormous volumes of multi-temporal remote sensing data on a nationwide scale.
Each year in Canada, thousands of hectares of farm fields and forests are transformed into different land-use classes, most often for industrial or urban development. These land-use changes occur continually in the vicinity of metropolitan areas due to construction of new homes, shopping centres and office parks, but it is happening to some extent across the nation.
At 9 million square kilometres, Canada is the second largest country by land area on Earth. The task of assessing changes in land use is a complex one that falls upon the Agriculture, Forestry and Land Use Section, one of several groups within PIRD that tracks human activities affecting net CO2 exchanges with the atmosphere.
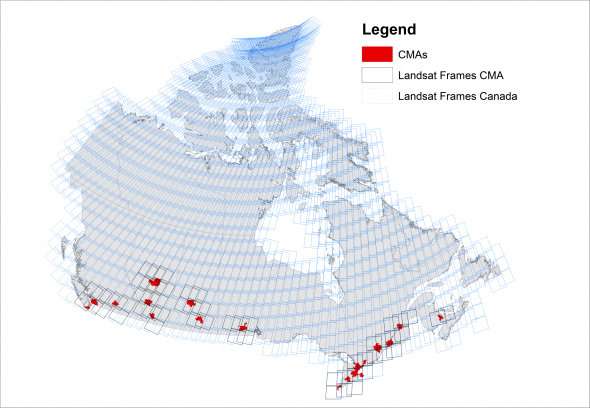
Canada’s Census Metropolitan Areas are priority areas for the PIRD.
PIRD, which is a division of Environment and Climate Change Canada (ECCC), aggregates these calculations into Canada’s annual National Greenhouse Gas Inventory Report (NIR) for submission each 15th April to the United Nations as a part of Canada’s commitments under the United Nations Framework Convention on Climate Changes (UNFCCC).
Quantifying the impact of land-use change on the greenhouse gas inventory is complicated, explained Doug MacDonald, PhD, manager of the Land Use Section. When agricultural land is developed into a new residential community, for example, a certain volume of CO2 sequestered in the soil is emitted into the atmosphere.
On the other side of the equation, however, the new neighborhood built on the site will likely have grass, trees and shrubs – and to some degree, these will contribute to sequestration. The net emissions and sequestrations of greenhouse gases for the developed area, therefore, will vary depending on the type of land-use change that occurred.
“We have to understand not only what areas changed, but what they changed from and what they become afterwards,” said MacDonald. “What the land becomes influences the total carbon balance.”
Remotely sensed data, mostly from Earth observation satellites, is one of the resources used to detect and evaluate land that has been converted from forest or farm fields into something else.
“The biggest challenge is monitoring change in a land sector the size of Canada,” said MacDonald. “It’s difficult to capture all the diverse changes that happen across the Canadian landscape.”
PIRD has teamed with PCI Geomatics, a Toronto-based provider of geospatial software, to develop an operational methodology based on Open Data Cube technology to perform multi-temporal change detection analysis on stacks of satellite images spanning decades. With a goal of scaling the technique to cover the entire country, PIRD and PCI have initiated a pilot, focused on land-use analysis in the Toronto area, where rapid urban development has been ongoing for more than 30 years.
Multi-temporal Analysis in the Open Data Cube
The application of algorithms to detect and classify changes in two satellite images captured at different times over the same area of interest is one of the most common uses of remotely sensed data. While extremely valuable in many projects, change detection analysis of two images has traditionally provided a snapshot of land-use or land-cover conversion at only a single point in time…until recently.
“The availability of entire archives of Landsat imagery from the U.S. Geological Survey and Sentinel data from the European Space Agency at no charge shifted the focus of change detection to multi-temporal analysis of many data sets,” said Arnold Hougham, vice president at PCI Geomatics.
The affordability of obtaining numerous satellite images, he explained, complemented by improvements in compute power and artificial intelligence algorithms pushed multi-temporal change detection to the forefront of geospatial research. Geoscience Australia, an office within the Australian federal government, developed a groundbreaking system, now known as the Open Data Cube, capable of four-dimensional analysis of imagery stacks.
The concept behind the Open Data Cube system was that multiple satellite images acquired over the same geographic area in time periods varying from days to months to years could be analyzed comprehensively for change. Instead of detecting a single instance of land-use or land-cover change between two dates, algorithms traverse through the time series and identifies when and where changes occurred, and also classifies the types of change.
The algorithms, for example, might pinpoint 10 different alterations in land use for the same parcel over two decades, depending on the temporal frequency of image collections stored in the data cube.
Because the Open Data Cube is designed to handle large volumes of raster data, its application in change detection projects involving land-use analysis covering expansive geographic regions and spanning many years is ideal for activities like PIRD’s. But preparing image data for Open Data Cube analysis presents challenges of its own.
Preparing Imagery for Analysis
The power of multi-temporal image processing in the Open Data Cube comes from its ability to detect change by performing pixel-to-pixel analysis. But for this to yield accurate results, each pixel in the stack of images must be precisely aligned so that pixels representing the same point on the Earth’s surface are compared with each other in imagery captured at different times.
“If you don’t geometrically align the pixels [among images in the stack], the algorithms inside the data cube will find misregistration instead of actual change,” said Hougham. “Standard orthorectification does not geometrically align pixels sufficiently for Open Data Cube analysis.”
In addition – and even more challenging – removing radiometric distortions among and within images must also be performed prior to processing to ensure reflectance values are consistent. Variations in sun illumination and atmospheric conditions can create differences between images collected at different times of the year. Shadowing effects can even introduce distortions from one side of an image to the other. These radiometric variations must be removed.
“As with geometry, if you don’t eliminate these atmospheric effects, data cube algorithms will detect radiometric differences instead of reflectance differences in the pixels,” he said.
PCI saw the opportunity to add value to this crucial aspect of multi-temporal analysis. A strength of its commercial GXL geospatial software package is its ability to process large catalogs of satellite imagery and create seamless mosaics covering extensive regions.
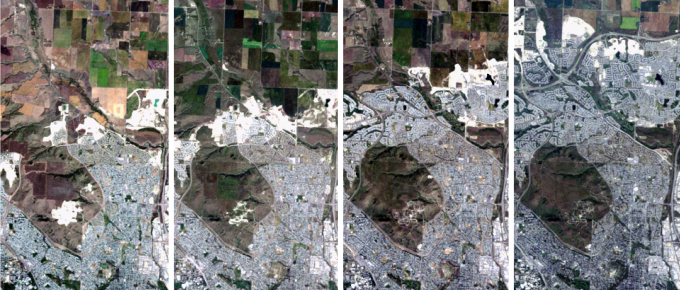
Urban expansion over the Calgary CMA (from left to right: 1984, 1990, 2001, 2009).
Leveraging this large volume processing capability, PCI experts used GXL as the platform for developing new algorithms to remove geometric and radiometric distortions from optical and Synthetic Aperture Radar (SAR) data sets and align pixels for analysis in the Open Data Cube. These processed data sets are called Analysis Ready Data (ARD).
Multi-temporal Analysis of Toronto
In the first phase of the PIRD pilot begun in early 2019, geospatial scientists from the Land Use Section and PCI fine-tuned the preparation of data for Open Data Cube analysis. Thirty years of Landsat 5 and 7 TM multispectral imagery acquired over the Toronto area were obtained and processed into ARD formats by personnel from both organizations using the new methodology. As the project moves into operational phases, this data preparation work will be performed entirely within PIRD.
Currently, the pilot has progressed to the analysis phase where multi-temporal change detection algorithms are being applied to the Landsat data stacks within the Open Data Cube. The most significant results produced so far have come from the Continuous Change Detection and Classification (CCDC) algorithm developed at the University of Connecticut by Dr Zhe Zhu.
The CCDC algorithm is proving adept at finding and quantifying land-use and land-cover change that results from physical disturbance as well as changes in condition. A physical land disturbance could be caused by development, but a change in land condition might be a result of vegetative stress or pest infestation, which can also impact the CO2 emission/sequestration balance.
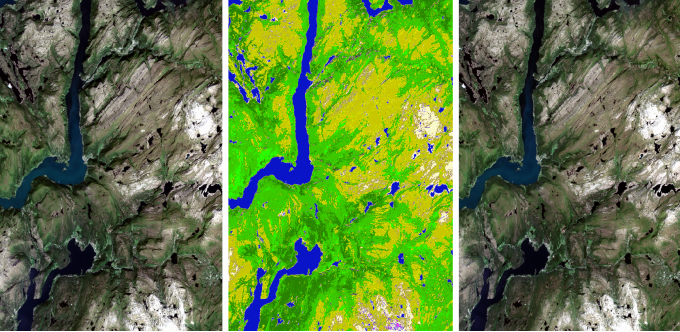
Conversion of raw satellite imagery to Analysis Ready Data using PCI Geomatics ARD Tools (from left: RGB, Spectral based pre-classification, topographically normalized ARD image).
As PIRD continues to scale the process up to an operational level on a nationwide scale, participants are optimists about results so far.
“[Results] are positive…It’s early days,” said PIRD’s MacDonald. “But this is the type of analysis we are going to need as we go forward capturing land-use change at a finer resolution across Canada.”
Detecting, identifying and quantifying land-use change at the detail of individual pixels in a satellite image is one of the most significant advantages of employing the Open Data Cube technology. The other is speed, according to MacDonald. Without the automated multi-temporal algorithms, much of the analysis would have to be performed by human interpretation, which would consume far more time and introduce the errors and inconsistencies of manual processes.
The next step for the Agriculture, Forestry and Other Land Use Section is to expand the pilot to other growing urban areas in Canada. Within PIRD, other sections are conducting similar pilots to apply multi-temporal change analysis to their areas of responsibilities. If all goes as planned, the technique will ultimately be rolled out on a nationwide basis in support of the annual greenhouse gas inventory.